Rachael Tappenden
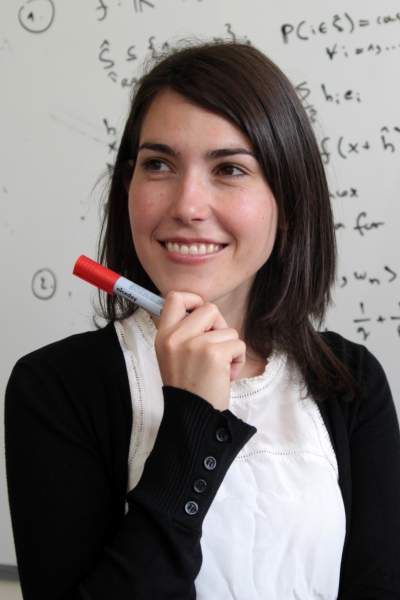
Lecturer
School of Mathematics and Statistics
Te Whare Wānanga o Waitaha
University of Canterbury
Private Bag 4800
Christchurch 8140, NEW ZEALAND
Room 714, Erskine Building
Telephone: +64 3 364 2987 ext 7687
Fax: +64 3 364 2587
Email: rachael.tappenden@canterbury.ac.nz
Research Background
I am a lecturer for the School of Mathematics and Statistics at the University of Canterbury, Christchurch, New Zealand. I was awarded my PhD from the University of Canterbury, where I worked under the supervision of Assoc. Prof. Ian Coope, and co-supervision of Dr Peter Renaud and Mr Bob Broughton. Following this, I became a Postdoctoral Researcher in the School of Mathematics at the University of Edinburgh, Scotland, where I collaborated with Dr Peter Richtarik, and also with Prof. Jacek Gondzio and Dr Burak Buke. I then worked as a Postdoctoral Researcher in the Department of Applied Mathematics and Statistics at The Johns Hopkins University, USA, where I worked with Prof. Daniel Robinson.
Research Interests
My research interests lie in the broad area of numerical linear algebra and optimization.
Recently I have studied convex optimization for big-data/data science applications, and the role that first order (gradient based) methods play in tackling such large scale problems. This included research on coordinate descent methods, which are currently a hot-topic of research because they work extremely well in this niche. This is due to their simplicity, low memory requirements, and low computational cost. Further, they appear to be some of the only algorithms that can scale to problems of huge dimension and subsequently they are proving to be very successful in a big-data environment.
I have also considered the mathematics of signal and image processing. This included work on subset selection type problems arising in MRI, the development of first order algorithms for compressed sensing, and I have also worked on image reconstruction techniques for computed tomography.
I am also interested in numerical linear algebra type problems, including the solution of systems of linear equations, finding eigenvalues of large, sparse, symmetric matrices, and preconditioning among others.